Philip Clarke, Professor of Health Economics, Nuffield College, Oxford University.
For a decade, science has faced a replication crisis in that many of the results of many of the key studies are difficult or impossible to reproduce. For example, the Open Science Collaboration in 2015 published a paper involving a replication of 100 psychology studies that found that many replications produced weaker evidence for the original findings. A study in the journal Science reproducing 18 economic experiments soon followed and again found that up to one-third could not be reproduced. The question still to be answered is whether health economics faces a reproducibility crisis, and if this is the case, what do we do about it?
To fully understand the reproducibility crisis, one must look at the incentives for authors trying to publish scientific articles. It is only human nature to regard results that are perceived as positive or statistically significant as telling a better story than negative or non-significant results. A common manifestation is P-hacking which arises when researchers look to report effects that are deemed statistical significance above a threshold such as 0.05. A recent analysis of over 21,000 hypothesis tests published in 25 leading economics journals show this a problem, particularly with studies employing Instrumental variables and Diff-in-Diff methods.
An initiative editors of health economic journals took in 2015 aimed at reducing P-Hacking was to issue a statement reminding referees to accept studies that: ‘have potential scientific and publication merit regardless of whether such studies’ empirical findings do or do not reject null hypotheses’. This appears to have had some impact, but health economics faces a unique set of challenges. Often there are pressures to demonstrate that an intervention is cost-effective by showing that it falls below a predefined cost per QALY threshold, which produces what could be termed cost-effectiveness threshold hacking.
Beyond formal hypothesis testing, the widespread use of key health economic results such as EQ-5D value sets means reproducibility is likely to be extremely important, as the results of such studies become inputs into potentially 100s of other analyses. It is perhaps not surprising that one of the only replications conducted in health economics has been over the EQ-5D-5L value set for England, although in less-than-ideal circumstances. Rather than a one-off, replication should be seen as integral to the development of foundational health economic tools such as value sets and disease simulation models that are critical to so much research.
The question now for the discipline is how can we promote and facilitate replication and avoid the pitfalls of P or threshold hacking?
One approach undertaken by the Mount Hood Diabetes Challenge Network has been to run comparable scenarios through various health economic diabetes simulation models. A recent challenge involved a comparison of 12 different Type 2 diabetes computer models that separately simulated the impact of a range of treatment interventions on Quality Adjusted Life Years (QALYs). The variation in outcomes across models was substantive, e.g. up to a six-fold variation in incremental QALYs associated between different simulation models (see figure 1). This suggested that the choice of simulation model could have a large impact on whether a therapy is deemed cost-effective and, when combined with threshold hacking, means that many economic evaluations are likely to be more in advocacy than science.
To create greater transparency, the Mount Hood Diabetes Challenge Network has also created specific guidelines for reporting economic evaluations that use diabetes simulation models to enable replication and has developed a simulation model registry. The registry is designed to encourage those developing models to provide documentation in one place and report on a set of reference simulations. Modelling groups are also encouraged to update these simulations each time the model changes, which provides a benchmark to compare different simulation models and how models evolve. Health economic model registries can potentially improve the science of economic evaluation in the same way clinical trial registries have improved the conduct and reporting of randomized controlled trials in medicine.
Finally, those conducting experiments or quasi-experimental methods can now submit registered reports. This initiative started with psychology journals with the idea that authors submit the protocol before undertaking the study to a journal for peer review. After review, if the registered report is accepted, the journal commits to publishing the full study regardless of the results’ significance. Registered reports are a way of avoiding the pitfalls of P-hacking and publication bias.
There are now more than 300 journals that allow registered reports, but take up by economic journals has been slow. Quality of Life Research and Oxford Open Economics are the only two options for those undertaking health economic experiments. Hopefully, these initiatives and an online petition signed by more than 145 health economists will encourage other health economic journals to provide this option in future.
Health economics embracing registered reports and developing health economic model registers are two ways to strengthen the discipline as a science.
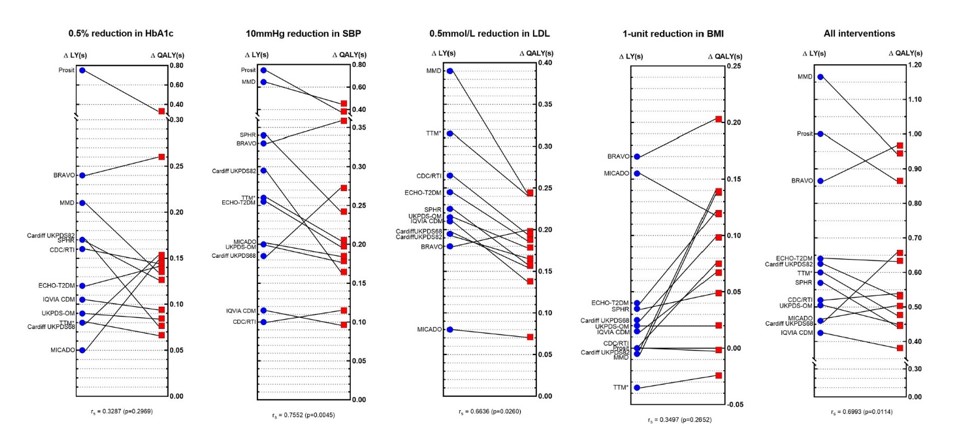
Figure 2 Comparisons of incremental life-years (ΔLYs) and incremental QALYs (ΔQALYs) across different models by intervention profile. Originally published Tew M, Willis M, Asseburg C, et al. Exploring Structural Uncertainty and Impact of Health State Utility Values on Lifetime Outcomes in Diabetes Economic Simulation Models: Findings from the Ninth Mount Hood Diabetes Quality-of-Life Challenge. Medical Decision Making. 2022;42(5):599-611. doi:10.1177/0272989X211065479 (Note figure is Creative Commons)